As hurricanes, tornadoes and other extreme weather events increase in frequency and severity, it is more important than ever to use the latest technology to improve and accelerate climate research and forecasting.
As the Atlantic hurricane season reaches its peak, NVIDIA Research today announced a new generative AI model, called StormCast, that emulates atmospheric dynamics with high accuracy, enabling reliable weather forecasts at the mesoscale — larger than a storm but smaller than a cyclone — which is critical for disaster planning and mitigation.
Stormcast, detailed in a paper co-authored by Lawrence Berkeley National Laboratory and the University of Washington, comes at a time when extreme weather events are claiming lives, destroying homes, and costing more than $150 billion a year in damages in the United States alone.
This is just one example of how generative AI is driving incredible advances in climate research and actionable extreme weather forecasting, helping scientists tackle some of our greatest challenges: saving lives and saving the world.
NVIDIA Earth-2 is a digital twin cloud platform that combines the power of AI, physics simulation, and computer graphics to simulate and visualize global weather and climate predictions with unprecedented accuracy and speed.
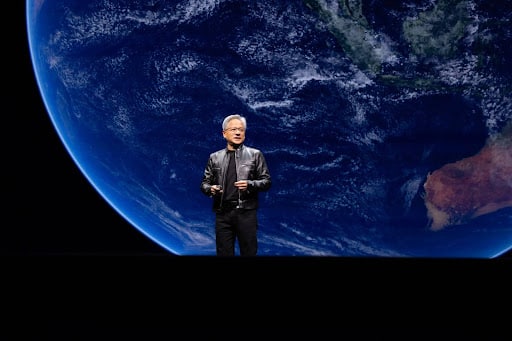
In Taiwan, for example, the National Research Center for Earth Science and Disaster Prevention uses CorrDiff, an NVIDIA-generated AI model available as part of Earth-2, to predict the fine scale of typhoons.
CorrDiff can super-resolve 25-kilometer-scale atmospheric data 12.5 times down to 2 kilometers, which is 1,000 times faster than previous methods and requires 3,000 times less energy for a single inference.
This means the center’s potentially life-saving work that previously would have cost approximately $3 million for CPUs can now be accomplished for approximately $60,000 on a single system with NVIDIA H100 Tensor Core GPUs — a significant reduction that demonstrates the energy efficiency and cost savings that generative AI and accelerated computing can bring.
The center also plans to use CorrDiff to predict downwash in urban areas, a phenomenon in which strong winds flow down to street level, damaging buildings and affecting pedestrians.
Now, StormCast has added hourly autoregressive forecasting capabilities to CorrDiff, allowing it to predict future results based on past results.
Local focus and global impact
Global climate research begins at the regional level.
Physical hazards due to weather and climate change vary greatly at regional scales, but reliable numerical weather predictions at this level are computationally expensive because high spatial resolution is required to represent the underlying hydrodynamic motions at the mesoscale.
Regional weather prediction models (also known as convection-permitting models, CAMs) have traditionally forced researchers to make a variety of trade-offs in resolution, ensemble size, and cost.
CAM helps meteorologists track the development and structure of storms, as well as monitor a storm’s convective mode – how a storm configures itself when it develops. For example, the likelihood of a tornado forming is based on a storm’s structure and convective mode.
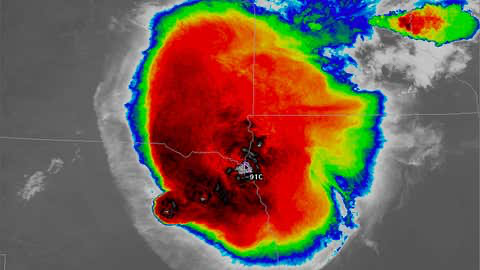
CAM can also help researchers understand the impacts of weather-related physical hazards at the infrastructure level.
For example, using global climate model simulations to inform CAM can help translate gradual changes in the water content of large atmospheric rivers into flood predictions in vulnerable coastal regions.
At lower resolutions, machine learning models trained on global data have emerged as useful emulators of numerical weather prediction models that can be used to improve early warning systems for severe events. These machine learning models typically have a spatial resolution of around 30 kilometers and a temporal resolution of 6 hours.
Now, with the help of generation diffusion, StormCast achieves this on a three kilometer, hourly scale.
Though the model is still in its early stages, when applied to precipitation radar it is already providing forecasts with lead times up to six hours, which is up to 10% more accurate than the National Oceanic and Atmospheric Administration’s (NOAA) state-of-the-art three-kilometer operational CAM.
Additionally, StormCast output exhibits physically realistic heat and moisture dynamics, predicting over 100 variables including temperature, moisture concentration, wind and precipitation radar reflectivity values at multiple closely spaced altitudes, allowing scientists to see realistic 3D variations in a storm’s buoyancy, a first in AI weather simulation.
NVIDIA researchers used NVIDIA accelerated computing to speed up calculations and train StormCast on approximately three and a half years of NOAA climate data for the central United States.
Further innovation will emerge
Scientists are already looking to exploit the model’s advantages.
“Given both the extraordinary impact of organized thunderstorms and winter precipitation and the enormous challenge of confidently predicting it, creating computationally tractable storm-scale ensemble weather forecasts is one of the great challenges in numerical weather forecasting,” said Tom Hamill, head of innovation at The Weather Company. “StormCast is a remarkable model that addresses these challenges, and The Weather Company is pleased to collaborate with NVIDIA on the development, evaluation, and future use of these deep learning forecast models.”
“Developing high-resolution weather models requires AI algorithms that analyze convection, which is a huge challenge,” said Imme Ebert-Uphoff, machine learning lead at Colorado State University’s Cooperative Institute for Atmospheric Research. “NVIDIA’s new research explores the possibility of achieving this with diffusion models like StormCast, taking a major step toward developing future AI models for high-resolution weather forecasting.”
Research breakthroughs like these, along with accelerated and visualized physically accurate climate simulations and digital twins of our Earth, show how NVIDIA Earth-2 is enabling a critical new era of climate research.
Learn more about sustainable computing and NVIDIA Research, a global team of hundreds of scientists and engineers focused on topics including climate AI, computer graphics, computer vision, self-driving vehicles and robotics.
Featured image courtesy of NASA.
Please see the software product information notice.