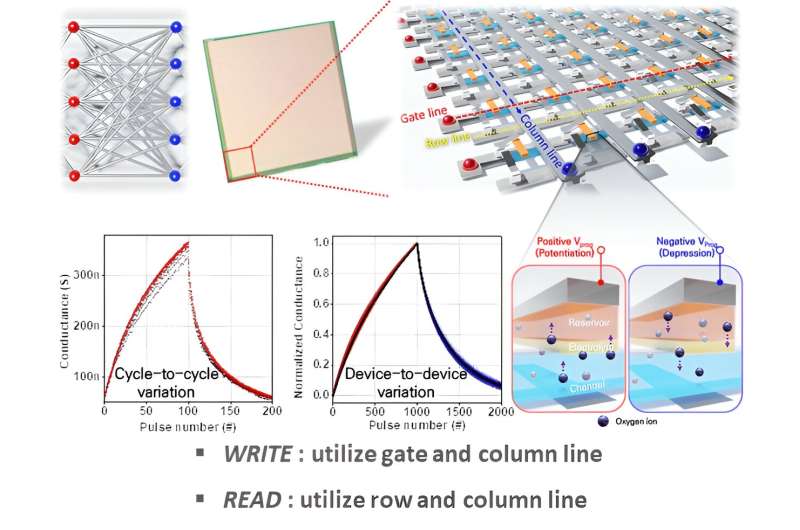
The cross-point array structure and operation of the three-terminal ECRAM device fabricated in this study (top). Measurement results of an array of three-terminal based electrochemical memory devices, showing excellent performance in both cycling and device-to-device variability, far exceeding the training requirements of the neural network (bottom). Credit: POSTECH
The research team demonstrated that analog hardware using ECRAM devices can maximize computational performance for artificial intelligence, demonstrating its commercial potential. The research was published in Science Advances.
Due to rapid advances in AI technology, including applications such as generative AI, the scalability of existing digital hardware (CPUs, GPUs, ASICs, etc.) is reaching its limits, stimulating research into analog hardware specialized for AI computation.
Analog hardware adjusts the resistance of semiconductors based on external voltage or current and utilizes cross-point array structures with vertically intersecting memory devices to parallelize AI calculations. Although it outperforms digital hardware in certain computational tasks and continuous data processing, it still struggles to meet the diverse requirements of computational learning and inference.
To address the limitations of analog hardware memory devices, a research team led by Professor Kim Se-young of the Department of Materials Science and Engineering and the Department of Semiconductor Engineering focused on electrochemical random access memory (ECRAM), which manages electrical conductivity through the movement and concentration of ions.
Unlike traditional semiconductor memories, these devices feature a three-terminal structure with separate paths for reading and writing data, allowing them to operate at relatively low power.
The research team successfully fabricated ECRAM devices using three-terminal based semiconductors in a 64 x 64 array. Experiments revealed that hardware incorporating the team’s devices exhibited excellent electrical and switching properties, with high yield and uniformity.
Additionally, the team applied the Tiki-Taka algorithm, a cutting-edge analog-based learning algorithm, to this high-yield hardware, successfully maximizing the accuracy of AI neural network training calculations.
In particular, the researchers demonstrated the impact of the “weight-preserving” properties of hardware training on learning and confirmed that the technique does not overload the artificial neural network, highlighting the potential for commercializing the technology.
This work is significant because the largest array of ECRAM devices for analog signal storage and processing reported in the literature to date is 10 × 10. The researchers have succeeded in implementing these devices at the largest scale possible, with different characteristics for each device.
“By developing large-scale arrays based on new memory device technology and developing analog-specific AI algorithms, we have identified the potential for AI computational performance and energy efficiency that far exceeds current digital methods,” said Professor Kim Saeyoung of POSTECH.
Further information: Kyungmi Noh et al., “Retention-aware zero-shift technique for Tiki-Taka algorithm-based analog deep learning accelerators,” Science Advances (2024). DOI: 10.1126/sciadv.adl3350
Provided by Pohang University of Science and Technology
Citation: Researchers develop next-generation semiconductor technology for artificial intelligence with high efficiency and low power consumption (August 1, 2024) Retrieved August 1, 2024 from https://techxplore.com/news/2024-08-gen-semiconductor-technology-high-efficiency.html
This document is subject to copyright. It may not be reproduced without written permission, except for fair dealing for the purposes of personal study or research. The content is provided for informational purposes only.