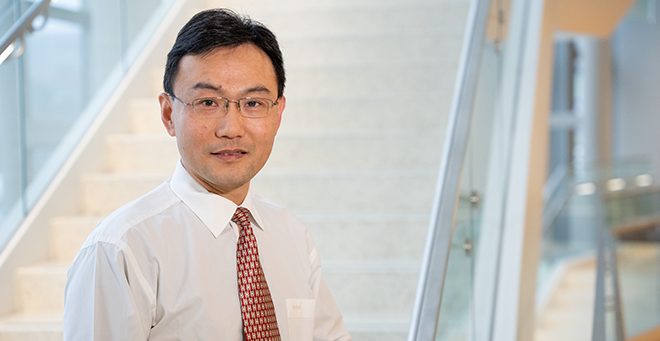
Photo: Brian Goodchild
Artificial intelligence (AI) can improve the diagnostic accuracy of images, predict patient outcomes from large datasets that can guide treatment plans, and analyze individual patient data to tailor interventions to individual needs. is already changing the way healthcare is delivered.
Massachusetts Chan College of Medicine researcher Feifang Liu, Ph.D., associate professor of population and quantitative health sciences, is researching another important application of AI: national efforts to advance health equity. It’s part of it.
In 2022, Dr. Liu was one of the first Leadership Fellows of the National Institutes of Health’s Artificial Intelligence/Machine Learning Consortium to Advance Healthcare Equity and Research Diversity (AIM-AHEAD) program. The program is a partnership between academics and researchers to strengthen their participation and representation. Improve underrepresented communities in the development of AI and machine learning models and increase the capabilities of this emerging technology to address health disparities and inequities.
“Feifan’s commitment to AIM-AHEAD and being part of this AIM-AHEAD structure is a step forward in not only the value of AI, but also the way we think about how AI works in healthcare systems and society as a whole. ,” said Ben S. Gerber, MD, MPH, a professor of population and quantitative health sciences who is working on several projects with Liu. “What are the risks? How do we address fairness, bias, trust, and other ethical issues in artificial intelligence?”
Liu is the principal investigator on two major research initiatives resulting from the AIM-AHEAD fellowship. The first, DETERMINE (Diabetes Prediction and Equity through Responsible Machine Learning), is a $1.4 million 2-fund partnership with the University of Illinois at Chicago and Temple University to develop AI-powered multivariable risk prediction models. Annual NIH AIM-AHEAD Consortium Development Grant. Integrate social, demographic, and clinical factors for accurate, unbiased, generalizable, and interpretable type 2 diabetes prediction. Dr. Gerber is co-principal investigator on the study, now in its second year.
“The main goal is to build a responsive AI model that predicts the risk of developing type 2 diabetes, and to evaluate how generalizable the model is across different institutions and how well the model can be used across different demographic subs. It’s about assessing how fair it works across groups,” Liu said. “We also conducted simulation analyzes to identify potential implications for real-world clinical practice, particularly improving access to preventive medicine and prevention programs for minorities disproportionately affected by type 2 diabetes. I will improve.”
At the heart of AI applications are the algorithms underlying machine learning models.
Existing clinical guidelines for type 2 diabetes prevention rely on a simplistic and inaccurate definition of prediabetes and rely on limited measures such as blood sugar levels and BMI, Liu explained. did. Researchers are integrating non-medical socio-economic data, including neighborhood, environmental and economic characteristics, into the DETERMINE algorithm to more accurately identify people at risk and direct the allocation of prevention and treatment resources. We hope that this will lead to fair distribution.
The second study, AI2Equity, is a $3 million, four-year grant funded by the National Heart, Lung, and Blood Institute in 2024. In partnership with OCHIN, a national community health network, and Temple University, a multidisciplinary team of researchers aims to: Build deep learning models that incorporate social determinants of health, structured electronic health records, and clinical notes to improve predictions of cardiovascular disease. According to Liu, this project provides a solid foundation for promoting equitable cardiovascular disease prevention.
This model will be compared with currently used cardiovascular risk prediction tools.
“For both projects, we will continue to assess and improve the generalizability and fairness of the models across different institutions and different settings,” Liu said. “To reduce bias, we develop training algorithms that ensure that information closely related to sensitive attributes, such as race and ethnicity, are excluded from training models.Research shows that AI This could unintentionally amplify the signal and exacerbate disparities for marginalized groups.Finally, we would like to show that the interpretability of this model can be improved and better support clinical decision-making. That’s what I think.
Liu and Gerber said the earlier and more accurately a person can identify their risk of developing diabetes or cardiovascular disease, the better their health outcomes will be by preventing or delaying the disease through lifestyle and medication. .