Summary: A new AI tool identified long-term COVID-19 infection in 22.8% of patients. This was a much higher rate than had been previously diagnosed. By analyzing extensive health records from approximately 300,000 patients, the algorithm is able to identify symptoms specifically associated with SARS-CoV-2 infection, rather than pre-existing symptoms, to combat the long-lasting novel coronavirus. Identify.
This AI approach, known as “precision phenotyping,” can help clinicians distinguish between long-term coronavirus symptoms and other health problems, potentially increasing diagnostic accuracy by about 3%.
Important facts:
AI-based precision phenotyping: Improve diagnostic accuracy by identifying long-term COVID-19 infections only after ruling out other causes of symptoms in health records. Broader representation: Algorithmic diagnoses reflect Massachusetts’ demographic profile and address biases found in traditional diagnosis codes. Research Opportunities: Algorithms have the potential to advance the future. Research on the genetic and biochemical factors of long novel coronavirus subtypes.
Source: Harvard University
Previous diagnostic studies suggested that 7 percent of the population was suffering from long-term COVID-19 infection, but a new AI tool developed by Army Gen. Brigham found that number to be much higher at 22.8 percent. It has become clear that.
AI-based tools can sift through electronic medical records to help clinicians identify long-term COVID-19 cases. This mysterious condition can include a series of persistent symptoms such as fatigue, chronic cough, and brain fog after SARS-CoV-2 infection.
The algorithm used was developed by extracting anonymized patient data from the clinical records of approximately 300,000 patients across 14 hospitals and 20 community health centers in the Massachusetts General Brigham System.
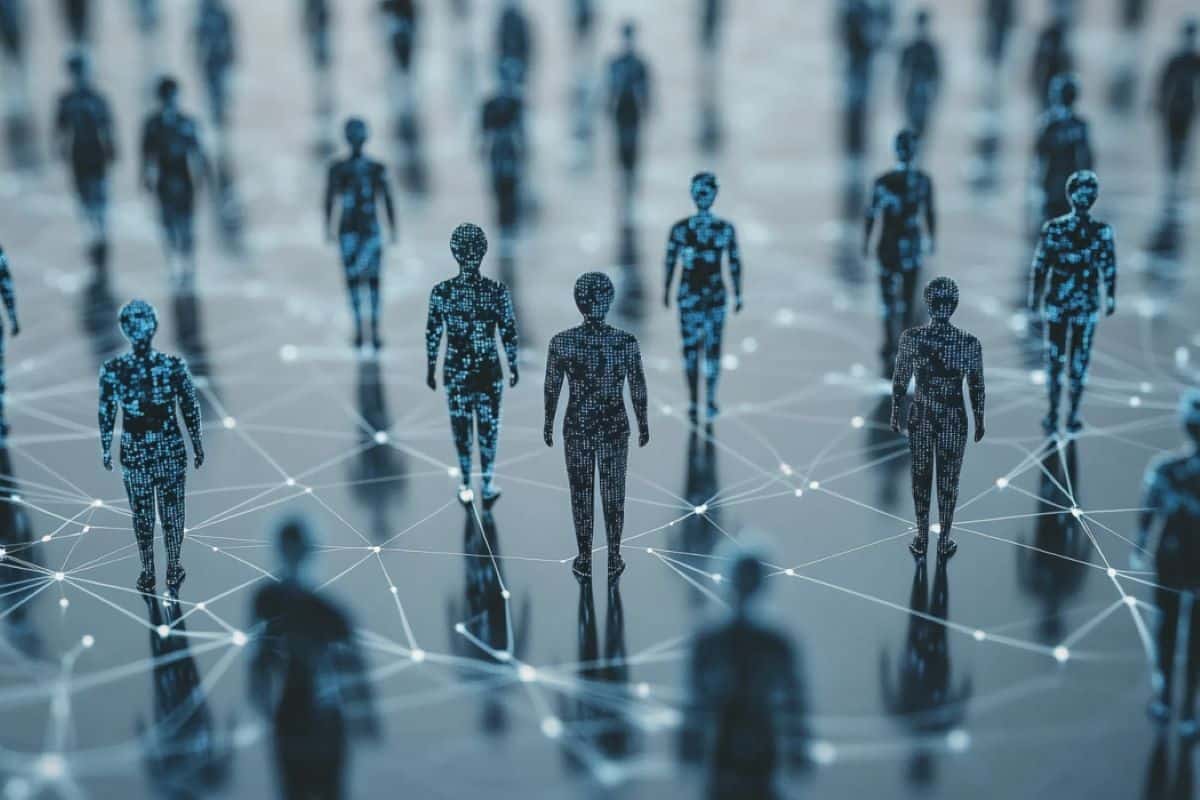
The results, published in the journal MedRxiv, could identify more people who should receive treatment for this potentially debilitating condition.
“Our AI tools can transform the foggy diagnostic process into something crisp and focused, empowering clinicians to understand difficult conditions.” said lead author Hossein Estiri, director of AI research at the Center for Information and Learning. MGB Healthcare System (CAIBILS) and Associate Professor at Harvard Medical School.
“With this study, we may finally know what long-term COVID-19 infections really are and, more importantly, how to treat them.”
For the purpose of the study, Estilli and colleagues defined long-term COVID-19 infection as a diagnosis of exclusion that is also associated with infectious disease. This means that the diagnosis could not be explained by the patient’s specific medical records and was related to COVID-19. In addition, the diagnosis had to have been ongoing for at least 2 months during the 12-month follow-up period.
A new method called “precision phenotyping” developed by Estiri et al. scrutinizes an individual’s records to identify symptoms and conditions associated with COVID-19 and to distinguish symptoms from other illnesses. Track symptoms over time.
For example, the algorithm can detect whether shortness of breath is due to a pre-existing condition such as heart failure or asthma, rather than a long-term COVID-19 infection. Only when all other possibilities have been exhausted will the tool flag a patient as having long-term COVID-19 infection.
“Physicians are often faced with the challenge of juggling a complex web of symptoms and medical histories, unsure of which threads to pull, while balancing busy caseloads. If we had the tools to do that systematically on behalf of our patients, that could be a game-changer,” said co-lead author and internal medicine resident at Brigham and Women’s Hospital, a founding member of the Massachusetts General Brigham Health System. A certain Arale Azir said. .
The new tool’s patient-centered diagnosis may also help alleviate bias built into current diagnosis of long-term coronavirus, researchers say, adding official ICD-10 diagnosis codes for long-term coronavirus pointed out that diagnoses tend to be directed toward patients with easier access to medical care.
The researchers said their tool was about 3 percent more accurate, yet less biased, than the data captured by ICD-10 codes. Specifically, their study found that unlike long-term coronavirus algorithms that rely on a single diagnosis code or individual clinical experience, individuals identified as long-term coronavirus patients reflect the broader population composition of Massachusetts. and demonstrated biasing results toward certain groups, such as those with greater access to health care. care.
“This broader reach ensures that marginalized communities, who are often excluded from clinical research, are no longer visible,” Estilli said.
Limitations of the study and the AI tools include that the health record data used by the algorithm to describe long-term coronavirus symptoms may be less complete than the data that doctors collect in clinical notes after a visit. It includes something.
Another limitation is that the algorithm did not capture possible worsening of previous conditions that may have been symptoms of long-term coronavirus. For example, if a patient had COPD and it worsened before developing COPD-19, the algorithm could have removed that episode, even if it was a long-term COVID indicator.
The decline in COVID-19 testing in recent years has also made it difficult to determine when a patient first became infected with COVID-19.
The study was limited to patients in Massachusetts.
Future studies may investigate the algorithm in cohorts of patients with specific conditions such as COPD or diabetes. The researchers also plan to make the algorithm publicly available in open access so that doctors and health systems around the world can use it on their patient populations.
In addition to opening the door to better clinical care, this study could lay the foundation for future research into the genetic and biochemical factors behind the different subtypes of the long-lasting coronavirus. be.
“Questions about the true burden of long-term COVID-19, questions that have remained elusive until now, now seem more within reach,” Estilli said.
Funding: Supported by the National Institutes of Health, National Institute of Allergy and Infectious Diseases (NIAID) R01AI165535, National Heart, Lung, and Blood Institute (NHLBI) OT2HL161847, and National Center for the Advancement of Translational Sciences (NCATS) UL1 TR003167. UL1 TR001881, and U24TR004111.
J. Hügel’s research was partially funded by a fellowship within the IFI program of the German Academic Exchange Service (DAAD), the Federal Ministry of Education and Research (BMBF), and the German Research Foundation (426671079).
About this AI and long novel coronavirus research news
Author: MGB Communications
Source: Harvard University
Contact: MGB Communications – Harvard University
Image: Image credited to Neuroscience News
Original research: Open access.
“Accurate phenotyping to curate a study cohort of patients with post-COVID-19 acute sequelae (PASC) as a diagnosis of exclusion” (Hossein Estiri et al.) MedRxiv
abstract
High-precision phenotyping to curate research cohorts of patients with acute sequelae of COVID-19 (PASC) as a diagnosis of exclusion
Scalable identification of patients with acute sequelae of COVID-19 (PASC) is hampered by the lack of reproducible and accurate phenotyping algorithms, suboptimal accuracy, demographic bias, and PASC. Difficult due to underestimation of diagnosis code (ICD-10 U09.9). ).
In a retrospective case-control study, we developed a highly accurate phenotyping algorithm to identify a study cohort of PASC patients defined as a diagnosis of exclusion. We used longitudinal electronic health record (EHR) data from more than 295,000 patients at 14 hospitals and 20 community health centers in Massachusetts.
This algorithm employs an attention mechanism to filter out aftereffects that can be explained by prior conditions. An independent chart review was performed to calibrate and validate the accurate phenotyping algorithm.
Our PASC phenotyping algorithm improves accuracy and prevalence estimates and reduces bias in identifying Long COVID patients compared to the U09.9 diagnosis code.
Our algorithm identified a PASC study cohort of over 24,000 patients (approximately 6,000 using the U09.9 diagnosis code) with 79.9 percent accuracy (compared to 77.8 percent with the U09.9 diagnosis code). did.
The estimated prevalence of PASC was 22.8%, which was close to national estimates in this region. We also provide a detailed analysis outlining clinical attributes, including identified residual effects by organ, comorbidity profiles, and temporal differences in PASC risk.
The PASC phenotyping method presented in this study boasts excellent accuracy, accurately measures the prevalence of PASC without underestimating it, and shows less bias in accurately identifying Long COVID patients. Show.
Our algorithm-derived PASC cohort serves as a starting point to delve into the genetic, metabolic, and clinical complexity of Long COVID, hampered by limited size and available outcome data. This study overcomes the limitations of recent PASC cohort studies.